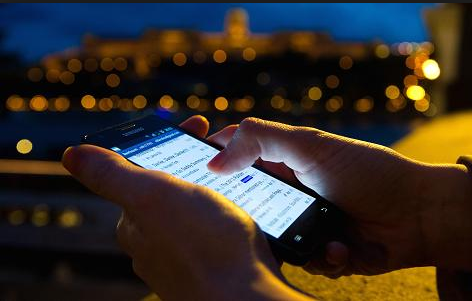
Data Science and Right balance of Digital Analytics Can Save Traditional Banking and Insurance!
As the fintech revolution takes over the financial and insurance industry data science, and the right balance of digital analytics can improve and save the traditional banking and insurance. The bellow research by Dataiku shows some critical areas where data science can improve banking and insurance.
Banks are currently storing more than 1 exabyte of data. How can this information be effectively used to solve the challenges facing today’s financial institutions? We thought it might be interesting to create a visual that tells the story.
Traditional companies in banking and insurance can use data science to survive the era of internet giants and financial technology startups. Big data is in its early days and as blockchain and IOT take over the financial industry will have to manage better data science and the way to correlate with their customers and ecosystems.

Over the course of many centuries, the banking and insurance industries have developed processes, products and infrastructures that have shaped the economic structure of humankind. But now, they are being challenged by industry outsiders who appeared on the world stage a mere couple of decades ago, and some who emerged just a scant few years ago, but who nonetheless are already rewriting the rules of financial services. These challengers include Internet-era giants like Google, Amazon, Facebook, Apple, Baidu and Alibaba; nimble digital startups like Credit Karma, Lending Club, Square, Lemonade, TransferWise and GoFundMe; and even, through the Internet of Things, wholly unlikely competitors like manufacturers of consumer and industrial goods.
Ultimately, GAFA (Google, Amazon, Facebook, Apple) has a Big Data and algorithm advantage. However, banks and insurance companies can fight back by accelerating their digitization path and utilizing their own advantages in big data and data science. Specifically, traditional banks and insurers can leverage existing assets that give unique advantages over non-traditional challengers in their industries, namely:
1. Untapped Reservoirs of Customer Data – With data mining and predictive analytics, the hidden value in the first of these assets – massive stores of day-to-day transactional customer data – can provide a unique advantage in better understanding, predicting and delivering what customers want, while helping to better address risk, fraud and market uncertainties.
2. An Extensive Branch / Office Network – The second asset, physical branch or office networks, can seem like merely a cost overhead, but it can play a vital role in developing meaningful customer relationships as financial services become increasingly digitized. As the online giants are learning, digital-only relationships have their limits: a binding customer experience is built on both physical and digital touchpoints.
Traditional banks and insurers are at an advantage here, if they make optimal use of their network to build relationships. Regional managers at companies like Bank of America and M&T Bank are, for example, seeing a real evolution as their physical branches morph into advising centers for customers, with one regional M&T manager noting a swing in service activity underway from 80% transactions and 20% expert advice to 20% transactions and 80% expert advice.
3. Stronger Customer Trust – The existing physical touchpoints for traditional financial services companies can also serve to reinforce an important third asset: trust. While the financial crisis did shake consumer confidence, individuals’ trust in traditional financial institutions remains strong. According to an IBM survey, 70% of respondents indicate that they trust traditional banks more than non-bank competitors, and when asked in another survey which institution they trust more to safeguard their personal information and privacy, consumers ranked traditional financial institutions higher by a wide margin over new online providers.
4. People with Quantitative Skills and Industry Expertise – Traditional financial service companies have a further advantage in having long employed professionals with advanced mathematical and statistical skills, providing them with a ready workforce of industry-savvy quantitative experts who can be trained to compete with GAFA and fintech on what has to date been their primary home field advantage: an adroit use of big data and algorithms to create great customer experiences in the digital, and increasingly the physical, spheres. In other words, banks and insurance companies are well-positioned to master data science.
Success depends on the speed with which traditional banks and insurers respond to these new challengers, in their skillful exploitation of their competitive assets, and in assembling the right people, data, tools and processes to get the job done.
Equipped with the right people, processes and tools, traditional banks and insurance companies can not only avoid the fate of becoming the backend plumbing for GAFA and fintech challengers, they can appropriate the advantages of these newcomers and merge them with their own to become the new marketplace innovators of the 21st century.
To learn more about how Data Science can play a role in traditional Banking and Insurance download the free whitepaper from Dataiku here: http://pages.dataiku.com/advanced-analytics-for-banking-and-insurance2-0
About Dataiku
Dataiku develops Dataiku Data Science Studio, the unique data science platform that enables companies to build and deliver their own data products more efficiently. Thanks to a collaborative and team-based user interface for data scientists and beginner analysts, to a unified framework for both development and deployment of data projects, and to immediate access to all the features and tools required to design data products from scratch, users can easily apply machine learning and data science techniques to all types, sizes, and formats of raw data to build and deploy predictive data flows.